Imagine a world where your voice commands are instantly understood and executed, where transcriptions are generated in real-time with impeccable accuracy, and where language barriers are a thing of the past. ASR speech recognition technology is making this vision a reality, transforming the way we interact with machines and access information. In this comprehensive guide, we will delve into the power of ASR speech recognition technology, its evolution, key components, deep learning techniques, real-world applications, challenges, and crucial factors to consider when selecting an ASR solution.
Table of Contents
Key Takeaways
Explore the power of Automatic Speech Recognition (ASR) technology in 2023, which has evolved from Bell Labs’ “Audrey” to current deep learning advancements.
ASR offers increased efficiency and faster adaptation over traditional transcription methods with key components including acoustic models, language models and decoders.
Real world applications include customer service, healthcare and education. Challenges such as background noise can be addressed through advanced techniques like signal processing or data augmentation.
Exploring ASR: Automatic Speech Recognition Defined
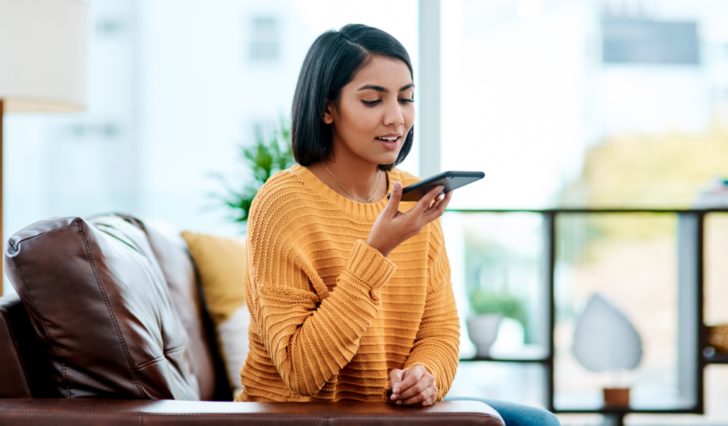
Automatic Speech Recognition (ASR) is a revolutionary speech recognition technology that enables the conversion of spoken language into written text. Through the use of advanced machine learning algorithms like deep learning approaches and neural networks, ASR systems, including speech recognition systems and automatic speech recognition software, have the capacity to process human speech accurately and provide real-time transcripts. These systems have evolved from simple directed dialogue conversations to natural language conversations, incorporating Natural Language Processing (NLP) to enhance generated transcripts with punctuation and capitalization.
Industries and applications from customer service and contact centers to healthcare and education have been profoundly impacted by ASR technology. The capabilities of modern ASR systems extend beyond just converting speech to text; they can also understand multiple languages, dialects, and accents, as well as differentiate speakers in conversations. This versatility and adaptability make ASR an invaluable tool in today’s fast-paced, multilingual world.
The Evolution of ASR
The journey of ASR technology dates back to 1952 when Bell Labs developed “Audrey,” a digit recognizer that marked the beginning of voice recognition technology. Over the years, researchers continued to advance ASR capabilities, and by the 1970s and 1980s, they started utilizing statistical models and machine learning algorithms to enhance the accuracy of speech recognition systems. Hidden Markov Models (HMMs) became a prevalent approach for modeling speech patterns and were extensively used in ASR systems.
In the 1990s, the introduction of neural networks marked a significant milestone in ASR technology. Recurrent Neural Networks (RNNs) and Long Short-Term Memory (LSTM) networks proved to be highly effective in modeling sequential data like speech. This led to a noticeable increase in ASR system accuracy.
Fast forward to recent years, advancements in deep learning and the availability of vast amounts of training data have further augmented ASR performance. Deep Neural Networks (DNNs) and Convolutional Neural Networks (CNNs) are now routinely used in ASR to extract high-level features from speech signals.
ASR vs. Traditional Transcription
When compared to traditional transcription methods, ASR offers several advantages, including increased efficiency, speaker differentiation, and faster adaptation to various accents and terminology. ASR technology can distinguish between voices in human speech during conversations, lectures, meetings, and proceedings, providing valuable insights into who said what.
Moreover, ASR systems can quickly assimilate information from a variety of related documents, enhancing their recognition capabilities for different accents, dialects, and terminology. This adaptability makes ASR suitable for interactive voice response systems and other applications that require accurate, real-time transcription.
Key Components of ASR Systems
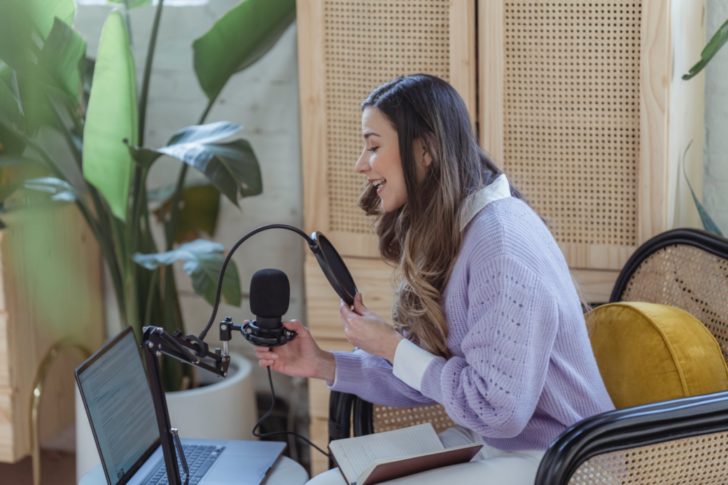
At the heart of every ASR system are three primary components: acoustic models, language models, and decoders. These components work together to accurately transcribe speech by analyzing the audio signal, predicting the likelihood of word sequences, and generating the final transcription.
Proceeding further, we’ll delve into the specific roles of each component in the ASR process.
Acoustic Models
Analyzing the audio signal and identifying phonemes – the smallest units of sound in a language, acoustic models serve a vital function in ASR systems. These models are responsible for converting raw audio data into a representation that can be further processed by the ASR system.
Modern ASR systems employ advanced neural acoustic models, such as:
QuartzNet
CitriNet
ContextNet
Conformer-CTC
Conformer-Transducer
These models help improve the accuracy of computer speech recognition, making them essential for the development of speech recognition applications, including speech recognition software and voice commands.
The output of the acoustic model in a deep learning speech recognition pipeline is the probability of characters or words at each time stamp. By accurately identifying and processing the phonemes in the audio signal, acoustic models lay the foundation for the subsequent components of the ASR system – language models and decoders – to build upon.
Language Models
Language models, also known as language model systems, are statistical models employed to estimate the likelihood of a sequence of words in a given language. They are trained on vast corpora of text data and can be utilized for various natural language processing tasks, such as speech recognition, machine translation, and text generation. In the context of ASR systems, language models are used to estimate the probability of word sequences, thereby aiding in the resolution of homophones and enhancing transcription accuracy.
The benefits of utilizing language models in ASR systems include improved accuracy by disambiguating homophones and providing contextual information for words. Additionally, they can minimize the amount of data required for training an ASR system and reduce the training time. However, obtaining the necessary data to train language models and the computational expense of running them may impede their use in real-time applications.
Decoders
Decoders are the final component of ASR systems that bring together the outputs of acoustic and language models to generate the final transcription. They directly convert the summarized audio into characters, ensuring that the transcription accurately represents the original spoken words.
By combining the strengths of both acoustic and language models, decoders play a critical role in ensuring the overall accuracy and effectiveness of ASR systems.
Deep Learning Techniques in ASR
Deep learning techniques have revolutionized the field of ASR, leading to improved accuracy and performance across various applications. These techniques enable ASR systems to better understand.
dialects
accents
context
multiple languages
This makes them invaluable tools in today’s interconnected world.
Moving forward, we’ll examine the benefits of applying deep learning in ASR and consider some prevalent deep learning architectures in the field.
Advantages of Deep Learning in ASR
Deep learning offers numerous benefits in the realm of ASR, including:
Enhanced comprehension of dialects, accents, context, and multiple languages
Significant increase in ASR accuracy when compared to traditional methods
Capability of deriving complex patterns and relationships from speech data
Models such as deep neural networks (DNNs) and recurrent neural networks (RNNs) have demonstrated these benefits and have contributed to the advancement of ASR technology.
Furthermore, deep learning models possess the capability to scale up to process large amounts of data and perform parallel processing, making them suitable for real-time and large-scale ASR applications. This scalability, combined with their ability to learn from extensive datasets and demonstrate strong generalization to unseen examples, provides robustness when dealing with variations in speech, such as different accents and dialects.
Popular Deep Learning Architectures
Several deep learning architectures are popularly used for ASR, including Connectionist Temporal Classification (CTC), Listen, Attend and Spell (LAS), and Recurrent Neural Network Transducer (RNNT). These architectures enable end-to-end training without the need for forced alignment or separate language models, simplifying the ASR pipeline and reducing the requirement for handcrafted features.
By utilizing these advanced architectures, ASR systems can achieve greater accuracy and performance, making them more effective in a wide range of applications.
Real-World Applications of ASR Technology
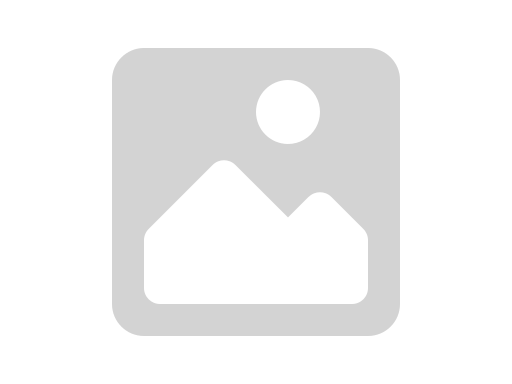
ASR technology has found numerous real-world applications that are transforming industries such as customer service, healthcare, and education. The ability to accurately transcribe and analyze spoken language in real-time has opened up new possibilities for creating more efficient and accessible systems.
As we move on, we’ll highlight some of the foremost applications of ASR technology.
Customer Service and Contact Centers
ASR technology is pivotal in the customer service sphere, enhancing call center efficiency, offering real-time recommendations, and facilitating conversation analysis for quality assurance. By accurately transcribing phone calls and providing instant insights, ASR technology enables customer service representatives to better understand and address customer needs.
Additionally, ASR can be used to automate certain tasks, such as routing calls to the appropriate department or providing answers to frequently asked questions, further enhancing the efficiency of contact centers.
Healthcare
Healthcare is another sector where ASR technology is having a significant impact. ASR can be utilized to transcribe medical records, facilitate communication between patients and healthcare providers, and support telemedicine services.
This not only increases the productivity and precision of healthcare processes, but also improves patient-provider communication and enables the development of voice-activated healthcare applications.
Education
In the educational sector, ASR technology offers numerous benefits, including providing live captions for lectures, assisting students with disabilities, and enabling voice-controlled learning tools. By transcribing lectures in real-time, ASR enables students to better understand and engage with the material, while also providing an accessible learning experience for students with disabilities.
Furthermore, the integration of ASR technology into language learning platforms allows for real-time feedback on pronunciation and fluency, enhancing the learning experience.
Overcoming Challenges in ASR Implementation
While ASR technology offers numerous benefits, it is not without its challenges. Background noise and variations in accents and dialects can affect transcription accuracy, making it essential to develop and implement robust solutions that can overcome these obstacles.
Next, we’ll examine the particular challenges related to background noise and accents and dialects, along with potential solutions to mitigate them.
Background Noise
Background noise can significantly interfere with ASR systems’ ability to accurately recognize speech, as they may have difficulty distinguishing between noise and speech. To address this challenge, advanced noise reduction techniques, such as signal processing techniques and deep learning methods, have been developed to improve the performance of ASR systems in noisy environments.
These techniques can be used to reduce the amount of background noise in the audio signal, allowing for the reduction of background noise in the audio signal.
Accents and Dialects
Accents and dialects can pose challenges for ASR systems, as they may not be able to correctly recognize words spoken in different accents or dialects. To overcome this challenge, it is crucial to develop models that can adapt to diverse speech patterns.
This can be achieved through data augmentation techniques, transfer learning, and the employment of multi-lingual models, which can help ASR systems better understand and transcribe speech in various accents and dialects.
Choosing the Right ASR Solution
Selecting the right ASR solution is a crucial decision that can impact the effectiveness and ease of use of the system. Factors to consider when choosing an ASR solution include:
Evaluating accuracy
Customization options
Integration capabilities
Privacy considerations
In what follows, we’ll probe into each of these elements and discuss their significance in choosing the perfect ASR solution.
For more info, visit Thinking of using open-source Whisper ASR? Here are the main factors to consider
Evaluating ASR Accuracy
ASR accuracy is typically measured by word error rate (WER), which calculates the number of errors in a transcription compared to the original audio. Evaluating ASR accuracy is vital for:
Assessing the performance of the system
Identifying areas for improvement
Ensuring that the system can accurately transcribe speech in various contexts and for different speakers.
By measuring the WER of different systems, researchers and developers can ascertain which system performs better and make informed decisions about which system to use or further develop.
Customization and Integration
Customization and integration options are important factors to consider when selecting an ASR solution, as they can impact the system’s effectiveness and ease of use. Customization options include language and dialect adaptation, acoustic model tuning, and domain-specific vocabulary, while integration options encompass integration with other applications, such as customer service and contact centers, healthcare, and education.
By considering these factors, users can select an ASR solution that is both powerful and adaptable to their specific needs and requirements.
Privacy Considerations
Privacy considerations are crucial when implementing ASR technology, as sensitive information may be transcribed and stored, requiring robust data protection measures. To ensure the protection of user data, it is essential to:
Evaluate data privacy
Obtain user consent
Assess data storage and retention
Ensure encryption and security
Verify compliance with applicable regulations
By taking these factors into account, users can select an ASR solution that prioritizes privacy and security without compromising performance and accuracy.
Summary
In conclusion, Automatic Speech Recognition (ASR) technology has come a long way from its humble beginnings as a simple digit recognizer. Today, ASR systems harness the power of deep learning and advanced algorithms to accurately transcribe speech in real-time across various industries and applications. While challenges such as background noise and variations in accents and dialects persist, innovative solutions are continuously being developed to overcome these obstacles. By carefully considering factors such as accuracy, customization, integration, and privacy, users can select the right ASR solution that suits their specific needs, paving the way for a more connected and efficient future.
Frequently Asked Questions
What is ASR in speech recognition?
Automatic Speech Recognition (ASR), commonly referred to as simply ASR, enables us to interact with computer interfaces using our voice in a manner that closely resembles normal conversation.
What is the difference between ASR and NLP?
ASR converts spoken language into text, while NLP allows computers to understand the meaning of natural language and thus provide accurate outputs.
Both technologies fall under Artificial Intelligence.
Is ASR the same as speech-to-text?
Yes, Automatic Speech Recognition (ASR) and Speech-to-Text (STT) are the same tasks, as they are both methods used to transcribe audio into text.
ASR is a critical component of speech AI, which enables humans to converse with computers through voice.
How does asr work?
ASR is an automated speech recognition system that utilizes voice recognition software to convert human speech into text. For decades, engineers and researchers have worked hard to develop these types of systems, making them increasingly powerful and accessible.
What are the key components of ASR systems?
ASR systems consist of acoustic models, language models and decoders that work together to effectively convert speech into text.